The Importance of Annotation in Machine Learning for Business Success
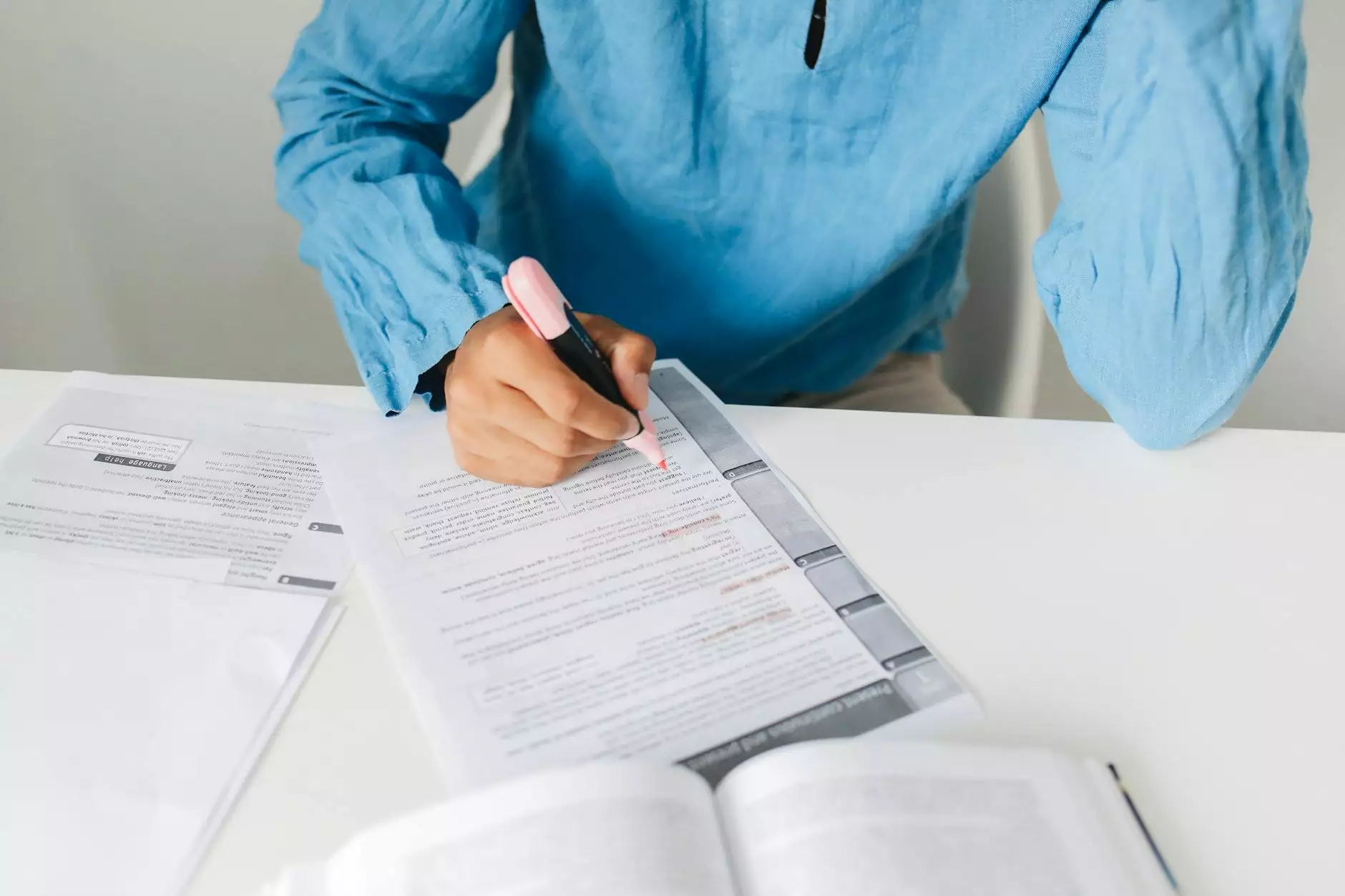
In today's fast-paced and ever-evolving business landscape, understanding and implementing advanced technologies is crucial for staying ahead. One such technology that has been making significant waves in the business realm is annotation in machine learning. This process is pivotal to enhancing data quality, improving customer experiences, and ultimately driving business growth. In this article, we will delve deep into the relationship between annotation in machine learning and its implications for businesses, specifically in the home services and keys & locksmiths sectors.
What is Annotation in Machine Learning?
At its core, annotation in machine learning involves the labeling of data to aid machine learning algorithms in understanding and processing that data more effectively. This can include:
- Image Annotation: Tagging elements in images for visual recognition applications.
- Text Annotation: Highlighting and labeling sections of text for natural language processing.
- Audio Annotation: Transcribing and tagging audio clips for speech recognition tasks.
With high-quality annotated data, machine learning models can learn patterns and make predictions more accurately, reducing error rates substantially.
The Role of Machine Learning in Home Services
In the home services industry, machine learning is transforming how businesses operate. Companies are leveraging annotated data to enhance services like:
- Customer service: By analyzing customer inquiries and complaints through annotated text data, companies can identify common issues and automate responses.
- Service recommendations: Using annotated images to recognize home features in customer uploads, businesses can recommend tailored services efficiently.
- Resource management: Annotated data helps in optimizing scheduling and resource allocation for home service providers.
These applications not only streamline operations but also enhance customer satisfaction, allowing businesses to build lasting relationships with clients.
How Annotation Enhances Keys & Locksmiths Services
For the keys & locksmiths industry, incorporating annotation in machine learning can significantly impact various business facets:
- Image Recognition: Annotating images of different locks and keys enables accurate identification and faster service delivery.
- Fraud Detection: Annotating transaction data helps locksmith companies in identifying fraudulent activities, protecting businesses and customers alike.
- Inventory Management: Annotated data on available stock can optimize inventory management systems, ensuring that popular services face no delays.
These improvements not only enhance operational efficiency but also improve safety and customer confidence in locksmith services.
Challenges in Annotation for Machine Learning
Despite its benefits, the process of annotation in machine learning does present challenges:
- Resource Intensity: Annotating large datasets requires considerable time and skilled labor.
- Quality Control: Inconsistent annotations can lead to poor model performance. Implementing strict quality assurance practices is necessary.
- Technological Integration: Ensuring that annotation tools integrate seamlessly with existing systems is crucial for maximizing efficiency.
Businesses must proactively address these challenges to fully leverage the advantages of machine learning.
Strategies for Effective Annotation in Machine Learning
To ensure the success of annotation in machine learning, businesses can adopt the following strategies:
- Invest in Training: Providing training for employees handling data annotation is critical. This ensures higher quality annotations that improve the performance of machine learning models.
- Utilize Advanced Tools: Leveraging state-of-the-art annotation tools can streamline the process and improve accuracy.
- Implement a Review Process: Regularly reviewing annotated data ensures quality and consistency, allowing for better model training.
- Collaborate with Experts: Partnering with data science professionals can help businesses design robust annotation frameworks tailored to their specific needs.
By implementing these strategies, businesses can maximize the efficacy of their machine learning models and drive better results.
Real-World Applications of Annotation in Machine Learning
Many companies across various sectors are already reaping the benefits of annotation in machine learning. Here are some notable examples:
1. Improving Customer Experience in Home Services
Several home service businesses are using annotated data to enhance customer experience. For instance, AI-powered chatbots that utilize annotated text data can understand customer queries accurately, providing quick and effective responses. Moreover, using annotated images allows these businesses to visualize what customers are dealing with, offering more tailored solutions.
2. Streamlining Operations for Locksmiths
Locksmith services have started employing machine learning models trained with annotated data to predict peak service times based on historical data. This predictive analytics capability allows businesses to better manage their workforce and efficiently allocate resources during high-demand periods.
3. Enhancing Security Measures
Both the home services and locksmith sectors utilize machine learning for improved security measures. By analyzing patterns in annotated transaction data, companies can identify unusual activities, helping to mitigate risks associated with fraud.
Future Trends in Annotation and Machine Learning for Businesses
The future of annotation in machine learning looks promising, with several trends emerging:
- Automated Annotation Tools: The rise of automated annotation tools will likely decrease the resource burden on businesses, enabling quicker model training.
- Integration of AI: As machine learning becomes more sophisticated, AI will play a larger role in analyzing and annotating data, providing insights beyond traditional methods.
- Real-time Data Annotation: The demand for real-time data processing will drive innovations in live data annotation, making machine learning models more reactive and adaptive.
These trends will enable businesses to harness the full power of machine learning, facilitating growth opportunities and enhancing service delivery in competitive markets.
Conclusion
In conclusion, annotation in machine learning is a cornerstone of successful business strategies in the home services and keys & locksmiths sectors. By investing in quality data annotation processes, companies can significantly improve their operational efficiency, customer satisfaction, and ultimately, their bottom line. As businesses continue to explore and adopt machine learning technologies, understanding the critical role of data annotation will be essential in navigating the future landscape of business intelligence.
To stay competitive, businesses should continuously innovate and integrate advanced data strategies that involve annotation in machine learning. With the right approach, the potential for growth and customer satisfaction is immense, shaping the future of industries and driving value for businesses and customers alike.